Feature-based indoor positioning with automatic incremental world model update
Feature-based indoor positioning system (FIPS) employs location-relevant signals of opportunity (e.g., signals transmitting from WLAN APs) for position estimation and has attracted attention both from academia and industry because it utilizes the widely existing infrastructure (e.g., WLAN, mobile phones) and requires no specific hardware deployment for positioning. Generally, an FIPS consists of the two stages
- offline stage for reference fingerprint map (RFM) construction and,
- online stage for position determination.
The main bottleneck which constrains the widespread commercial application of FIPS is the heavy workload to build the radio map and keep it up-to-date for large regions of interest (e.g., throughout a mall, covering an entire airport). An innovative approach which offers a framework to formulate and tackle the problems related to FIPS including RFM construction/update, positioning and trajectory tracking on the same basis is proposed in this PhD project.
First, an FIPS is deployed utilizing the existing WLAN signals within the HIL building of ETH, Zurich. The deployment of the FIPS includes: i) the collection of the RFM kinematically using the custom-developed mobile application with the help of an accurate external positioning system, which is used for the purpose of acquiring the reference data and validating the indoor positioning performance; and ii) the construction of RFM using a foot-mounted inertial measurement units (IMUs) for mimicking the process of crowd-sourcing-based RFM collection. This established FIPS is used as the testbed for experimental investigations.
Second, on the basis of the testbed, an fingerprinting-based indoor positioning approach specifically regarding the reduction of the online positioning computational complexity is proposed. The reduction is achieved by two treatments. One is modified Jaccard index-based coarsely positioning, which reduces the search space for refining the position estimation. This coarse position is capable of constraining the search space to a fixed level, which is independent of the size of the region of interest (i.e. the coverage of the FIPS). Another is relevant feature selection (e.g., using LASSO or adaptive forward-backward greedy search) for handling the situation of redundant number measurable features. It is thus determined which subset of features contributes more for achieving high quality positioning. This feature selection procedure is carried out at offline stage (for reducing the online storage complexity) and the online refining positioning uses only those relevant features to reduce time complexity.
Following-up from previous work of feature selection, compound dissimilarity measure (CDM) is proposed for addressing the case of variant number measurable features at different locations, which is a missing point of feature selection and makes the typical feature-based positioning methods (e.g., kNN) fail to work without a specific treatment. The idea of CDM is to compute the dissimilarity, the core for inferring the position from the feature space, between two measurements by dividing the computation into three parts according to the measurability of the features and weighting each part proportionally. The CDM enables the positioning even in case of the total of number of features in the region of interest is unknown, which is the situation of using the opportunistic location-relevant signals as features for indoor positioning.
Investigations suggest, that a focus is on detecting the changes (e.g., disappeared or new signals) occurred in the FIPS and incremental update the RFM according to the detected changes in the specific part of the region of the interest is vital. The change detection is designed for identifying the confidence level whether a given feature has changed via constructing an approximate hypothesis testing using bootstrap techniques. Once the changes have been detected, RFM update criteria, such including the new features into the RFM, eliminating disappeared features from the RFM or adjusting features values, are applied. In this way, the FIPS is capable of adaptively evolving by automatically keeping the RFM up-to-date.
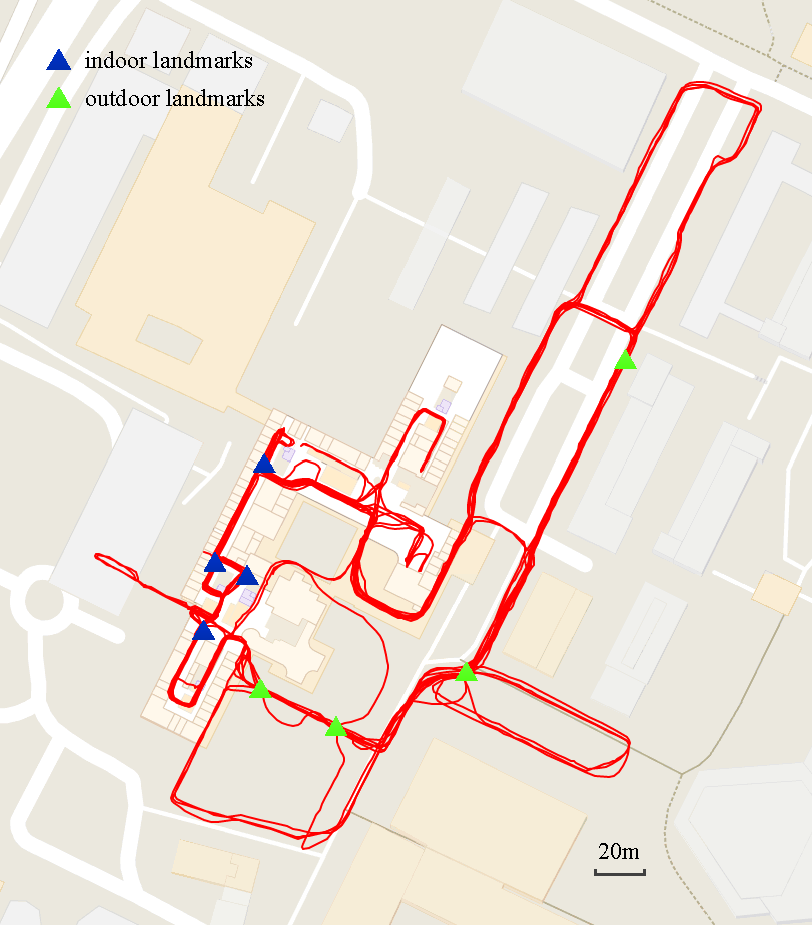
Contact
- Location location_onHIL D 47.2
- Phone phone+41 44 633 05 55
- Emailemailandreas.wieser@geod.baug.ethz.ch
- contactsvCard Download
Geosensorik und Ingenieurgeodäsie
Stefano-Franscini-Platz 5
8093
Zürich
Switzerland